1. INTRODUCTION
With upcoming activity tracking technologies, self-knowledge through collected data (also known as self-quantification, self-tracking) rapidly increases in the domain of health and fitness, making users’ abilities to self-regulate more and more important. According to Lupton (2016, p. 5), “digital data about people’s lives are also vital in their effects [...] they have begun to play a significant role in influencing people’s behaviors...” Users of these technologies get the possibility and authority to quantify, monitor, and analyze the collected data by using activity tracking technologies anywhere and anytime (Lupton, 2014, p. 77). Activity tracking technologies enable users to collect fitness and health-related metrics such as counted steps, burned calories, heart rate, and sleep cycles. Here, the term activity tracking technology refers to activity trackers, also called fitness trackers (e.g., wearables of Fitbit, Xiaomi, Garmin), smartwatches (e.g., Apple Watch, Samsung Galaxy Watch), and mobile applications (e.g., Strava). With all forms, it is possible to digitally log health and fitness-related metrics. While some activity trackers enable the possibility to automatically track health and fitness-related metrics with less effort, some mobile applications need the data to be manually logged by users, such as nutrition applications (Rooksby, Rost, Morrison, & Chalmers, 2014).
Especially in the human-computer interaction discipline, activity tracking technologies are investigated as a subtype of the so-called personal informatics systems. According to Li, Dey, and Forlizzi (2010), personal informatics systems enable people to receive information about themselves towards self-reflecting and developing self-knowledge about different personal areas. Furthermore, Li et al. (2010) differentiate between a system-driven and user-driven personal informatics system. Following the definition by Li et al. (2010), in this investigation activity tracking technologies will be characterized as a ‘mixed’ personal informatics system. Even if the activity trackers are mainly system-driven (collecting data through sensors and pedometers, and visualizing data automatically), users have yet the possibility to choose which of the functionalities they would like to use and to change default goals.
By now, activity tracking technologies draw the interest of different research fields (e.g., computer science, human-computer interaction, information science, system sciences, engineering, medicine, and social sciences). All in all, the publications indexed by Scopus increased from about 100 in 2015 to more than 450 in 2019 (Fig. 1A). Drawing on the systematic literature review of Shin et al. (2019), all publications after 2012 were included.
Fig. 1.
(A) Time series of publications on “activity tracking” 2013-2019. (B) Studied activity tracking devices. Source: Scopus (May 3, 2020); search in article titles, abstract, and keywords.
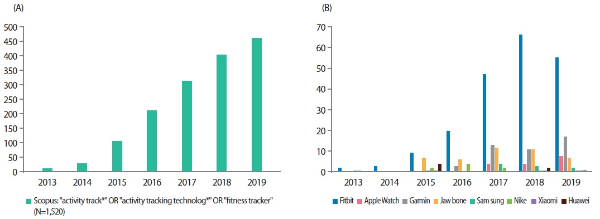
In particular, the research field of medicine and healthcare is interested in investigations about activity tracking technologies’ accuracy, reliability, feasibility, and validity (e.g., Diaz et al., 2015; Evenson, Goto, & Furberg, 2015; Martin et al., 2015; Rosenberger, Buman, Haskell, McConnell, & Carstensen, 2016). Besides this, to what extent and why users of activity tracking technologies engage, adapt, and use these devices was investigated as well (e.g., Fritz, Huang, Murphy, & Zimmermann, 2014; Gouveia, Karapanos, & Hassenzahl, 2015; Lyall & Robards, 2018; Nelson, Verhagen, & Noordzij, 2016; Rooksby et al., 2014). Additionally, there are also studies that investigated barriers and reasons why users stop using one or find workarounds (e.g., Harrison, Marshall, Bianchi-Berthouze, & Bird, 2015; Shih, Han, Poole, Rosson, & Carroll, 2015). Even if investigations show useful insights such as why and how participants use activity trackers, studies focusing on the use of information are yet rare. Mostly the emphasis is set on the functionalities and design of a system. To the best of our knowledge, there are only a few studies which are mainly focused on the users and interaction and engagement with the collected data, which reflects a major aspect of information science (Feng & Agosto, 2017, 2019a, 2019b; Feng, Li, & Agosto, 2017).
Particularly concerning the characteristics of personal informatics systems and self-quantification, we assume that the information provided by these technologies might play a crucial role in reflecting on and evaluating behavior and in learning more about oneself. Activity tracking technologies are mainly system-driven, which makes it crucial to investigate if the provided information is easy to use and easy to understand, as well as necessary in the eyes of the users. Since activity tracking technologies allow interpretation and evaluation, their correct understanding depends on the users, their knowledge, and their skills. Therefore, to better understand the use of the information, it is also crucial to understand if people can transform the provided information into actions, which in turn is dependent on the usefulness of the provided information. The perceived usefulness of information is crucial regarding health information literacy. As Stock and Stock (2013) already mentioned, users are differently engaging with information systems and the use of information systems depends on their level of information literacy. The more that users are information literate, the more they are able to interact with information systems. And the more information systems are usable, the more users will interact with them.
Therefore, apart from the system-side provision of information by an activity tracking device, this investigation aims to gather further useful insights from the perspective of information science, drawing on the subfield of information behavior.
Health information behavior and the tracking of someone’s activity is a rather new topic in information science. For many scientists, information behavior is more than searching and finding information, as for example information production and consumption behavior on information services (Friedländer, 2017a, 2017b; Scheibe, Fietkiewicz, & Stock, 2016). According to Wilson (2000, p. 49), “[i]nformation [b]ehavior is the totality of human behavior in relation to sources and channels of information, including both active and passive information seeking, and information use.” According to Pingo and Narayan (2019, p. 506), “[t]he understanding of how people make meaning out of fitness tracker data is a vital aspect of their information seeking, which provides an important and interesting perspective for information behaviour research.” Activity tracking technologies offer visualized data (graphs) and explanations on sleep cycles and heart rate zones. Is the provided information necessary and useful and, even more importantly, are users able to reflect on and adapt their behavior based on these explanations? According to Stock and Stock (2013), characteristics such as recognizing an information need, the ability to evaluate information, and the synthesis of previous and new obtained information is assigned to the concept of information literacy.
Information behavior depends on the context. For this study, the context is found in activity tracking devices and applications. However, research on information behavior on activity tracking can be said to be in a nascent stage.
To restrict and therefore enable deeper insights into results as each device has specific, individually designed, and integrated functionality and explanations, this study focuses on wearables provided by Fitbit. The search in the database Scopus showed Fitbit is the most investigated wearable (Fig. 1B). Fitbit was founded in 2007 in San Francisco and develops wireless wearable technology to track health and fitness-related data. Fitbit has more than 27 million active users worldwide and shipped five million devices worldwide in the last quarter of 2018 (Liu, 2019).
This investigation aims to derive implications for future research in many ways: To what extent is the need on activity and health information satisfied with data provided by activity tracking technologies? Are there any ambiguities regarding the understanding of how to interpret and reflect collected data? Is the provided information necessary and useful? The paper will give a consolidated theoretical background covering the concept of information behavior and an insight into investigations on activity trackers. The Methods section reveals the developed web-based survey, its distribution, and the used quantitative approaches. This is followed by answering the research questions (RQs) and a discussion of the findings and implications.
2. LITERATURE REVIEW
In recent years there has been growing interest in understanding why and how users engage with activity tracking technologies to better understand how to design and develop wearables. Rooksby et al. (2014) revealed that reasons for using activity trackers are to track walking (e.g., developing awareness), physical exercise, food and drink (e.g., because of being interested in losing or maintaining weight), weight, size, and sleep patterns. Further, investigations on activity tracking technologies also enable users to understand how activity tracking technologies change users’ information practices (Pingo & Narayan, 2019). Even if activity tracking technologies have much functionality in common, they are not equally important as it depends on the intended goals. Furthermore, activity tracking technologies might not be only crucial regarding gaining awareness of somebody else’s activity level, but also “to provide structure and motivation to people who feel incapable of implementing their intention of exercising without support” (Gouveia et al., 2015, p. 1305).
Gouveia et al. (2015) developed a mobile application called Habito to track physical activity. They explain that in order to better determine the success or failure of behavior change, it is crucial to investigate to what extent users engage with specific features. They revealed that the motivation to engage with an activity tracker depends on the readiness of users to be physically more active. The authors point out that most of their participants were in a short engagement phase (less than 10 sec.), where they only screen values instead of intensive reviewing and engaging with information. They conclude that even if behavior change is characterized as gaining valuable knowledge about oneself, users are not interested in reflecting on past tracked data and, therefore, in changing their behavior (Gouveia et al., 2015). Users of activity tracking technologies apply the devices with different goals, some reasons being “directive tracking [e.g., goal-driven], documentary tracking [e.g., awareness, reflection, confirmation], diagnostic tracking [e.g., identification of connections between things, searching for an answer for situations (headache, bad sleep), analyzing data], collecting rewards, and fetishised tracking [purer interest in gadgets and technology]” (Rooksby et al., 2014, p. 1167). Lyall and Robards (2018) investigated how users of activity tracking technologies engage with tracked data by conducting interviews. They question how the interviewees use their data and create an added value. They provide three roles which characterize activity tracking technologies, namely tool (to reach a goal, to raising awareness, to monitor), toy (playful gadget), and tutor (guiding towards a healthier lifestyle). Additionally, activity tracking technologies are also used to monitor progress and one’s own goals, to decrease the level of intensity (Patel & O’Kane, 2015), and to control one’s health (Gowin et al., 2019).
There are investigations which studied the impact of wearables on users’ behavior (Fritz et al., 2014; Ilhan & Henkel, 2018). Fritz et al. (2014) investigated long-term users and how wearables influenced their physical activity. The study highlights that long-term users developed an awareness of their physical activity regarding different settings (routine and nonroutine activities). Participants of the study by Fritz et al. (2014) also indicated that even if they use their wearable, they pay less attention to the data as they already know their behavior patterns. Gouveia et al. (2015, p. 1309) come as well to the conclusion that “when individuals become more self-reliant, use is more and more marked by brief, reassurance-seeking, glance interactions.” Ultimately, activity tracking technologies can impact users’ behavior by, for example, taking more steps instead of an elevator to receive more steps (Fritz et al., 2014; Ilhan & Henkel, 2018).
A downside of activity tracking technologies exists to the effect that self-quantification might lead to obsessive measuring. Fritz et al. (2014) point out that their study revealed that participants focus on numerical goals and data to receive credits through tracking. Activities that might not be able to be tracked by devices were less attractive, and a user avoids them or does other activities where they might receive more credits (Fritz et al., 2014; Harrison et al., 2015). Lyall and Robards (2018, p. 115) affirm this downside as well by explaining that the “potential problem here is that self-tracking data displays may dictate meaning and, by extension, define the human actions they record.”
Summarized, several studies have been carried out to understand why users use activity tracking technologies and how. We agree with Feng et al. (2017) as well as Feng and Agosto (2019a, 2019b) that the personal information management (PIM) of activity tracking users was rarely investigated. They draw on the framework of PIM (Jones, 2007, 2012) and investigated the personal health information management of users of activity tracking technologies (Feng & Agosto, 2019a, 2019b; Feng et al., 2017). The results show that health and fitness-related needs such as achieving a better performance require an information need (Feng & Agosto, 2019a). Feng and Agosto (2017) surveyed users of activity tracking technologies to investigate how users engage with the personal health information. They confirmed that users who used the device for the long term would continue to use it, similar to the results of Gouveia et al. (2015). Feng and Agosto (2017) also underline that 74% of the participants use their tracker almost all the time. Feng et al. (2017) showed that steps, distance, calories, sleep, and heart rate were mainly provided by activity tracking technologies (more than by half of the users' activity tracker). According to Feng et al. (2017), the provided information includes different types of data: raw data directly generated by activity tracker sensors, as well as more complex and processed information such as routes or calories burnt during an exercise. During the study by Feng et al. (2017) participants (current users) mainly agreed that the charts, tables, and timelines of activity tracking technologies are clearly presented. The interviews conducted by Pingo and Narayan (2019) showed that the visualized data were easy to use as well.
Nevertheless, as people bring along different requirements (needs), experience, and knowledge, users’ contexts and the role that information plays in this situation is decisive (Lyall & Robards, 2018). Therefore, experiences regarding the information provided by activity tracking technologies might be perceived differently. Especially, if users of activity tracking technologies see the raw data or diagrams and explanation for the first time it might affect the experience as well. Furthermore, a study conducted by Maher, Ryan, Ambrosi, and Edney (2017) revealed that three out of 37 former users and seven out of 200 current users indicated that they have problems with interpreting collected and provided data by their activity tracking devices. Further, two former users explained that it was difficult to understand the provided information by activity tracking technologies and that the information was not important. Furthermore, even if activity tracking technologies enable users to gain self-knowledge and to self-regulate, investigations in the past also revealed challenges. Zhang, Schaub, Feng, and Sadeh (2019) stress that activity tracking technologies do not give specific suggestions on how to improve poor sleep. Further, Liang, Ploderer, and Chapa-Martell (2017) investigated Fitbit users and showed that the definition on how the sleep metrics are measured is not reasonable. Even if users might be able to gain self-awareness and monitor their tracked health and fitness-related metrics, these metrics might raise questions which are not answered by them.
Based on these narrowed insights, the study aims to investigate to what extent the different types of information provided by an activity tracking device enable users to achieve their needs and if they reflect on and adapt their behavior based on the provided information. For this purpose, the next section introduces the theoretical background of this investigation and frames the work.
3. THEORETICAL BACKGROUND
This study draws on the concept of information behavior to better understand users’ needs and how the information provided by wearables and mobile application supports the accomplishment of these needs. Information behavior reflects active as well as passive activities by people. According to Case and Given (2016, p. 6), information behavior (IB) also “includes the broader context of how individuals ‘deal with’ information in their lives, so accounts for situation, time, affect, culture, geography, and other contextual elements in understanding people’s IB.”
Wilson (2000) emphasizes that information behavior is made up of three sub-sets, namely information-seeking behavior, information searching behavior as a sub-set of information-seeking, and the information use behavior. Ultimately, “by information behaviour is meant those activities a person may engage in when identifying his or her own needs for information, searching for such information in any way, and using or transferring that information” (Wilson, 1999, p. 249). Investigations within the context of information science are mainly focusing on information searching behavior, “particularly concerned with the interactions between information user (with or without an intermediary) and computer-based information systems, of which information retrieval systems for textual data may be seen as one type” (Wilson, 1999, p. 263). Besides activities such as seeking and searching, the activity to use information does not only mean to apply information but also to ignore information (Case & Given, 2016). Overall, the concept of information behavior is a “nestled field” (Wilson, 1999, p. 263). Considering all the subsets and terms within the concept of information behavior it is important to define terms related to information behavior (Agarwal, 2018). According to Case and Given (2016), information need is the situation where an individual realizes that there exists a knowledge gap to reach a desired goal. In the context of information behavior, information-seeking describes “a conscious effort to acquire information in response to a need or gap in your knowledge” (Case & Given, 2016, p. 6). It is important to realize, while investigating or speaking about information behavior, that the concept of information behavior does not only consist of the seeking-behavior as people “may choose not to seek, or information may simply find them [...] before a person even realizes that they want to learn more” (Case & Given, 2016, p. 7).
Up to this point and building on Case and Given (2016), in this study we define information need as the knowledge gap to be able to reach desired goals. According to the previously introduced literature, we pre-define these needs as developing awareness, improvement, assessment, and identifying and interpreting differences and saliences of fitness and healthrelated activities. The question that arises here is, what is the information and the source and who is the actor?
According to Agarwal (2015, p. 4), an actor is defined as a “seeker, user or person who is looking for information or who finds information on something unexpectedly.” In this case, the users of activity tracking devices are the actors, and we assume that they are using the wearables because they have a need, such as improving their health and fitness-related activities. For this need, it is crucial to know the current activity status. Therefore, there might exist a knowledge gap, like not knowing how much they are walking. Here the information need is understood as the desire to see the count of steps. Without knowing that, a user might not be able to improve the level of physical activity. Information use describes both the use of the information (Agarwal, 2015; Line, 1974) and also the ignoring (Case & Given, 2016). In this investigation, looking at the provided logged metrics and graphs is understood as using the information. We already defined information use, information need, and information-seeking, but we did not specify the term information. According to Case and Given (2016), there does not exist a homogenous understanding of information. Buckland (1991, p. 351) characterizes information by dividing it into three types: “Information-as-process” (e.g., informing someone), “Information-as-knowledge,” and “Information-as-thing” (such as documents). According to Feng and Agosto (2019b), as the wearables produce the data, there is the speech of “information-as-thing.” In information science, “we analyze information as data and meaning (knowledge) in context” (Stock & Stock, 2013, p. 22). Wearables provide data to their users; and the users give them meaning and gain knowledge in the context of fitness and health information.
The types of information provided by Fitbit are differentiated regarding their richness of information and complexity (Fig. 2). Type 1 is the solely visual displaying of a value like the actual heart rate, the number of steps, distance, amount of sleep hours, and so on. According to Feng et al. (2017), this type is also characterized as “raw data.” Type 2 describes provided information that is more detailed and offers more associated and aggregated values (e.g., time series, summarized workouts). Type 3 offers explanations regarding the heart rate zones and sleep quality.
Fig. 2.
Information types provided by Fitbit: Raw data (Type 1), aggregated data (Type 2), and explanations (Type 3).
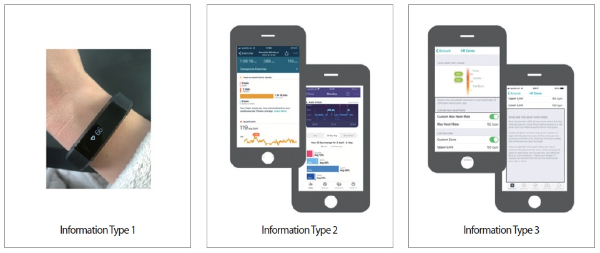
Especially regarding Information Type 3, we assume that users need to be able to understand how to reflect on and adapt the presented information and insights. The information behavior of users can be influenced by the usefulness of provided information by information services (Stock & Stock, 2013). Users who might be more information literate easily use and understand the information as opposed to people who are lower information literate. Therefore, health information literacy enters the stage. According to our context, we apply the definition of health information literacy as activity tracking technologies track health and fitness-related metrics. Eriksson-Backa, Ek, Niemelä, and Huotari (2012, p. 84) state that “[a] related concept that describes health-related information behaviour, including needs, seeking and use of information related to health or medicine is health information literacy [...].” Health information literacy is defined, according to the Medical Library Association (2005, p. 1), as abilities to “recognize a health information need; identify likely information sources and use them to retrieve relevant information; assess the quality of the information and its applicability to a specific situation; and analyze, understand, and use the information to make good health decisions” (Hirvonen, Pyky, Korpelainen, & Huotari, 2015, p. 3). Why is this important for this study? According to Hirvonen, Huotari, Niemelä, & Korpelainen (2012), the health information behavior research on physical activity showed that information needs and information use increase with higher task complexities and levels of activities.
Based on the theoretical foundation and previous investigations on activity tracking technologies, this paper aims to answer overall three RQs.
Based on the complexity of a need we assume that different needs require different types of information. For example, the need of interest or curiosity of knowing how many steps a user collected, what his or her actual heart rate is, or how many hours a user slept last night requires raw data (Information Type 1). On the contrary, complex activities such as getting to know which activities influence heart rate during a workout and, therefore, decisions on how to change a specific behavior require aggregated data (Type 2) and explanations (Type 3). Therefore, the first research question (RQ1) is formulated as follow:
-
RQ1: To What Extent Are Fitbit Users’ Needs Satisfied by Using the Provided Information (Type 1 and Type 2)?
-
Here we aim to oppose the two information types, the raw data (Type 1) as well as the aggregated data (Type 2), to better understand if the provided information is differently useful and if the information is also equally used. Generally, the use of diagrams needs engagement with the application and might provide summarized data over a period, while Type 1 needs regularly only a look at the wearable and shows real-time information.
-
RQ2: How Do Fitbit Users Perceive the Readability of Explanations about Heart Rate Zones and Sleep Stages’ Characteristics (Type 3)?
-
Fitbit provides explanations (Type 3). According to Nelson et al. (2016), the readability of information influences the engagement. Therefore, before we investigate if users can adapt and use that information (health information literacy), we wanted to examine the readability of this information (system-oriented).
-
RQ3: To What Extent Do Users Understand the Provided Explanations (Type 3) and How Do They Estimate Their Usefulness?
-
In the next step, we concentrate on the users and their use of explanations (Type 3). As Fitbit is using words and descriptions assigned to the health and fitness-related context, these explanations might not be self-explaining for users within the same context (e.g., seeing this explanation more frequently versus seeing them for the first time).
-
Further, according to the health information behavior and health information literacy concept, users need to have the abilities to “...assess the quality of the information and its applicability to a specific situation; and analyze, understand, and use the information to make good health decisions” (Hirvonen et al., 2015, p. 3). Therefore, we investigate if users know how to use these explanations to reflect on and adapt their behavior or if they will do so in the future.
4. METHODS
We conducted an explorative quantitative study in order to gain more insights from an information science perspective and constructed an online survey with the help of eSurveyCreator. com (Connaway & Radford, 2016). At the time of the survey, Fitbit’s newest function, the calculated sleep value, was not available. We decided to concentrate on the features steps (this is a minimum that all activity trackers provide), the heart rate, and sleep functions. Screenshots of Fitbit’s explanations contain information about the different sleep stages (light, deep, rapid eye movement [REM], awake) and heart rate zones (fat-burning, cardio-zone, maximum heart rate). For the screenshots included in the survey, Fitbit’s permission was acquired. The screenshots of Fitbit's sleep stages' characteristics were only for participants visible whose activity tracker offers the tracking of the heart rate.
The survey consists of four parts (see Supplemental Materials). The first part includes demographics and background questions (e.g., usage duration, exercise level) to gain first insights into the characteristics of the sample. The second part represents the investigations’ aim to understand the need for information to develop awareness, improve and assess health and fitnessrelated behavior, and identify and interpret differences and saliences and the information use (Type 1 and Type 2). The third part of the survey represents aspects that enable empowerment to change behavior positively by using Type 3. This part of the questionnaire includes readability statements adapted and modified from Nelson et al. (2016). Finally, the fourth part includes statements regarding health information behavior and the perceived usefulness and understandability of provided explanations. Those statements are connected with abilities assigned to the concept of health information literacy (such as being able to use, reflect on, or synthetize information). Therefore, this part mapped questions about reflecting on, adapting, and use of that information (Type 3), leaning on Eriksson-Backa et al. (2012) and the general definition of the Medical Library Association.
We segmented the third and fourth part of the survey into two parts―including users who already knew the explanations and users who saw the explanations for the first time during the survey.
The survey includes conditional questions to ensure that the participants of the survey only get questions based on the features they actually use. The survey also includes optional free-text fields to share further experiences. We developed two surveys, one in German and the other in English, which were distributed separately.
Before we distributed the survey, from March 28, 2019 until August 26, 2019, the questionnaire was pretested by six Fitbit users (two male and four female testers) and two volunteers for the translation. The language was slightly modified, and ambiguities were resolved. The questionnaire was distributed in diverse German and English-speaking health and fitness-related Facebook groups, Reddit, our own social network channels, universities (mailing-lists, face-to-face), and gyms. We did a convenience sample as it is not possible to reach all Fitbit users in the world. The convenience sample seems to be adequate for sharing the survey in Fitbit and fitness and health-related Facebook groups, and for other sources as they could include the target group. Therefore, the sample population was easy to contact. Participation was voluntary, and there were no payments or vouchers.
Data analysis was conducted with IBM Software SPSS Statistic 26, and variables as type string was coded into numerical values. Based on the variable ‘usage duration’ we created a new variable to summarize the characteristics into newbie (less than a week, 1-2 weeks, three weeks, a month), short-term (several months), medium-term (a year), and long-term (several years). Further, we grouped the birth cohorts into a new variable ‘Generation’ with Silver Surfers (born before 1960), Generation X (1960-1979), Generation Y (1980-1995), and Generation Z (born after 1995) (Fietkiewicz, Lins, Baran, & Stock, 2016). Statements based on a Likert scale (here a 5-point Likert scale) and the variables ‘exercise frequency,’ ‘usage duration,’ and ‘Generation’ are ordinal, which is why we need to use nonparametric tests. As the data is mostly ordinally scaled, we apply the median (Mdn) and the interquartile range. We used the Mann-Whitney U test, a rank-based nonparametric test, to determine statistically significant differences between independent variables (with two characteristics) and dependent ordinal variables regarding the distribution of the answers (Laerd Statistics, 2018). For interpretation, lower mean ranks indicate lower values regarding the evaluation (Likert scale) and vice versa. The U test (via SPSS Statistics Legacy Dialogs) was used to determine whether the distribution of answers regarding the perceived readability differs between users who already knew these explanations and users who saw it for the first time (RQ2). Further, for answering RQ3, we conducted the U test for all statements assessing the perceived usefulness and understandability of the provided information except for the statements such as (I adapted my behavior vs. I will adapt my behavior) and (I reflected my behavior vs. I will reflect my behavior), as these activities are different. In the description of results, MR is the mean rank, U is the test statistic, z value is scaled to the standard normal distribution of the U-value, n is the number of participants, and p is the probability rejecting the null hypothesis (H0), although it is true, thereby erroneously accepting the alternative hypothesis (H1). The following significance levels were determined: p-value≤0.05*, p-value≤0.01**, p-value≤0.001***.
All in all, 1,016 persons participated in the survey; finally, 631 valid cases were identified.
5. RESULTS
Table 1 gives an insight into the sample’s characteristics. The majority of the participants were female (88.1%), and most users were between 40 and 59 (48.3%) or between 24 and 39 (37.7%) years old. The majority of the participants answered that they are exercising 1-2 times (25.5%), or three or more times per week (38.5%). In conclusion, most participants in this sample were somewhat physically active, and most participants were long-term users. Furthermore, 85.3% are using their wearable throughout the whole day and night.
5.1. RQ1: To What Extent Are Fitbit Users’ Needs Satisfied by Using the Provided Information (Type 1 and Type 2)?
Table 2 shows predefined needs, namely awareness, improvement, assessment, and identification/interpretation, and summarizes the evaluation of users’ information needs and if the usage of provided information (Type 1 and Type 2) satisfies their information needs. Regarding the information need, Table 2 shows that users mainly agree that they are looking at the provided information (Type 1 and Type 2) because they want to gain awareness (e.g., to get information how many steps they walked, gaining awareness about routines, current heart rate while working or exercising, how long do they sleep, how did they sleep after exercising), to improve their health and fitness-related behavior (to get information about current heart rate to reduce or estimate if it is good, going to bed earlier, to take stairs more often, generally to walk more), and at least, to get information to be able to assess the tracked health and fitness-related metrics (e.g., did they walk a lot of steps, is their heart rate too low/high, was it a ‘good’ or ‘bad’ sleep). They also mainly agree that they are looking at the diagrams because they want to interpret and identify differences and saliences (Type 2).
Table 2.
Satisfied information needs and information use regarding steps, heart rate, and sleep
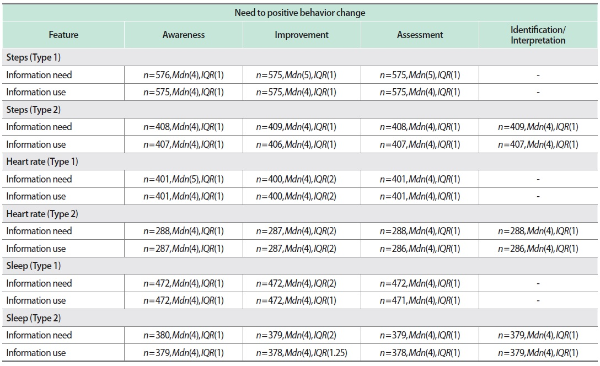
Type 1, raw data; Type 2, aggregated data; Mdn, median; IQR, interquartile range.
Table 2 confirms that participants mainly agree that the use of information, both ‘raw data’ (Type 1) regarding steps, heart rate, and sleep, and ‘aggregated data’ (Type 2), visualizing heart rate and sleep quality, satisfy their information needs. Notably, around 50% of the users agree (Mdn equals 4) that the tracked sleep diagrams (Type 2) help to identify and to interpret differences and saliences. Furthermore, Table 2 revealed that raw data regarding heart rate and sleep is more preferred than aggregated data. While 401 participants are viewing and using the heart rate as raw data (Type 1) to gain awareness, fewer participants are looking at the diagrams (N=288) and using them (N=287), even if they are mainly satisfied (Mdn equals 4).
5.2. RQ2: How Do Fitbit Users Perceive the Readability of Explanations about Heart Rate Zones and Sleep Stages’ Characteristics (Type 3)?
Table 3 shows the perceived readability of Fitbit’s heart rate zones explanation. The heart rate zones explanation includes information such as “Cardio (70-84% max heart rate) is the medium to high intensity exercise zone. In this zone, you are pushing yourself but not straining. For most people, this is the exercise zone to target”; or, “Zones are calculated based on a max heart rate of 220 bpm minus your age.”
Table 3.
Measured agreement or disagreement on readability regarding heart rate zones explanation
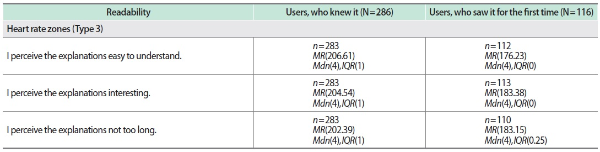
Type 3, explanations; MR, mean rank; Mdn, median; IQR, interquartile range.
The investigation differentiates between users who already knew the explanations and users who saw them for the first time. More than half of the participants who are consciously using the heart rate function did notice the listed explanation. Users who already knew the explanations as well as users who saw them for the first time mostly tend to agree (Mdn equals 4) that the readability is given (easy to understand, interesting, and not too long).
A Mann-Whitney U test was performed to determine if there were differences in the distributions of the answers (MRs) regarding the perceived readability. The distribution of the answer to the question whether the explanation about heart rate zones is easy to understand differs significantly (U=13410.000, z=-2.824, p≤0.01**). According to Table 3, the MR for users who already knew the explanations is higher (MR=206.61) than for the users who saw the heart rate zones explanations for the first time and perceived them as easy to understand (MR=176.23). This leads to the conclusion that participants who already knew the heart rate zones explanation have higher ranks (generally tend to agree stronger) than for users who saw them for the first time.
Table 4 shows the perceived readability of Fitbit’s sleep stages’ characteristics. The sleep stages’ characteristics explain, for example, “At night, your body cycles through different Sleep Stages. It usually moves from light sleep to deep sleep, back to light, then into REM, though sleep cycles vary naturally”; or, “You were [...] in Deep sleep. Deep sleep helps with physical recovery and aspects of memory and learning. If you’re feeling extra refreshed, you likely spent some solid time in this stage.”
Table 4.
Measured agreement or disagreement on readability regarding sleep stages' characteristics explanation

Type 3, explanations; MR, mean rank; Mdn, median; IQR, interquartile range.
Table 4 attracts attention as the number of participants who knew it is much higher (N=420) than in comparison to the heart rate zones explanation (Table 3). In contrast, the number of users who saw the sleep stages’ characteristics for the first time is smaller. Here, participants of both samples mainly agree (Mdn equals 4) that the readability of the sleep stages’ characteristics is present. Nevertheless, the applied U test revealed that the distributions of the answers, whether the information is easy to understand, interesting, and not too long differ significantly. Users who already knew the explanation tend to agree more (MR=235.07) than users who saw it for the first time (MR=163.91) that it is easy to understand (U=5612.500, z=-3.618, p≤0.001***). These findings behave similarly for finding the explanation interesting (U=5891.000, z=-3.192, p≤0.01**) and not too long (U=5733.500, z=-3.130, p≤0.01**). In both cases, the MR of users who knew it is higher than for users who saw the sleep stages explanation for the first time.
5.3. RQ3: To What Extent Do Users Understand the Provided Explanations (Type 3) and How Do They Estimate Their Usefulness?
Table 5 shows that participants, both those who already knew the heart rate zones explanation and users who saw them for the first time, are tending mainly to agree on the usefulness and understandability of the provided information. Both user groups mostly agree (Mdn equals 4) that the explanation is necessary and trustworthy. Furthermore, they also mostly agree that the explanation is sufficient and that they do not have further questions (Mdn equals 4), and that the explanation is not overloaded with information (Mdn equals 4). This observation is also applicable regarding understanding the explanations. Both user groups mainly agree that they learned new things (Mdn equals 4), know how to use the obtained information (Mdn equals 4), and understand how Fitbit is calculating the data (Mdn equals 4).
Table 5.
Perceived usefulness and understandability of heart rate zones explanation
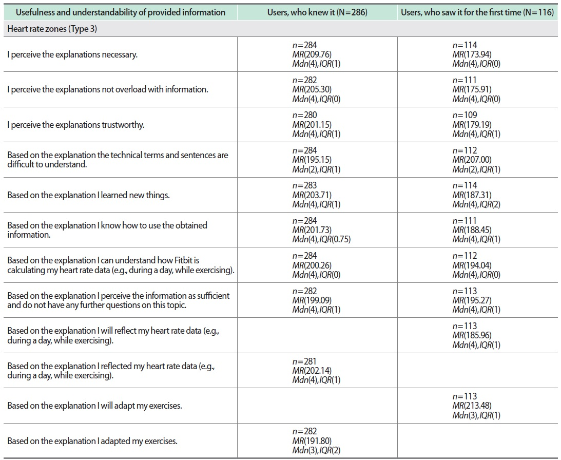
Type 3, explanations; MR, mean rank; Mdn, median; IQR, interquartile range.
Furthermore, both groups of users who knew it or got to know it during the survey mainly disagree (Mdn equals 2) that the technical terms and sentences are difficult to understand. According to the aspect of using the information to make good health decisions, users who knew it also mostly agree (Mdn equals 4) that they reflected on their behavior based on this explanation. Users tend to answer mainly neutral regarding the statement that they adapted their behavior (Mdn equals 3). The users who saw the explanation for the first time also mainly agree that they will both reflect on (Mdn equals 4) and adapt (Mdn equals 3, mode equals 4) their behavior based on the explanation in the future. Here, only the distributions of answers regarding the assessment are significantly different. The MR for users who knew the heart rate zones explanation is higher (MR=205.30) than for users who saw it for the first time (MR=175.91) regarding the statement that the information is not overloaded with information (U=13310.000, z=-2.697, p≤0.01**). Further, the distributions of the answers between the two groups also differ significantly regarding the statement that the explanation is necessary (U=13274.000, z=-3.144, p≤0.01**). The MR for users who already knew the heart rate zones explanation (MR=209.76) is higher than for users who saw them for the first time (MR=173.94).
Contrary to the heart rate zones explanation in Table 5, Table 6 shows two cases where the Mdn is different. Users who saw the sleep stages’ characteristics for the first time tend to be mostly neutral (Mdn equals 3) as opposed to users who already knew it and tended to mostly agree that the explanation is trustworthy (Mdn equals 4). This is similar regarding the understanding how Fitbit is calculating the data.
Table 6.
Perceived usefulness and understandability sleep stage characteristics' explanations
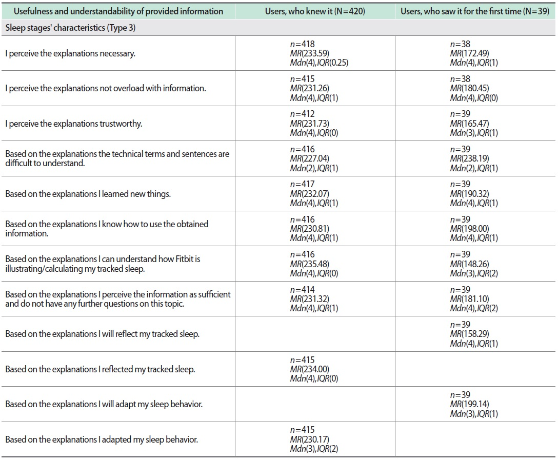
Type 3, explanations; MR, mean rank; Mdn, median; IQR, interquartile range.
Regarding the sleep stages' characteristics explanations, the MR and, therefore, the distributions of the answers for both types of users differ significantly regarding some aspects. Distributions of the participants’ agreement on the statements such as “I learned new things” (U=6642.500, z=-2.051, p≤0.05*), not overloaded with information (U=6116.000, z=-2.599, p≤0.01**), sufficient (U=6283.000, z=-2.449, p≤0.05*), understanding how the data is calculated (U=5002.000, z=-4.318, p≤0.001***), trustworthiness (U=5673.500, z=-3.299, p≤0.001***), and necessity (U=5813.500, z=-2.970, p≤0.01**) significantly differ between both groups. Regarding all these statements, the MR for users who already knew the sleep stage explanation is higher than for the other group, which revealed that they tend to agree stronger.
6. DISCUSSION
6.1. Fitbit Users’ Needs and Satisfaction Based on Using the Provided Information (Type 1 and Type 2) (RQ1)
The participants of this study mainly agree that they are using the three surveyed features (steps, heart rate, and sleep quality) of Fitbit. According to Feng and Agosto (2017), who reported that 74% of their participants use their tracker almost all the time, the sample in this study shows similar characteristics.
Most participants agree that they need to become more aware, improve their health and fitness-related behavior, and assess their behavior. Therefore, they agreed that they need the information provided by Fitbit. This investigation shows that the users in this study use the information provided by their activity trackers to meet their information need, and consequently, their health and fitness-related needs. These findings can be associated with Feng and Agosto (2017, 2019a) as they also found that users apply provided raw data and aggregated data displayed as diagrams. Furthermore, as the participants mostly agree that using the information enables them to satisfy their needs, this could indicate that results are easy to use and clearly presented. This is in line with Feng et al. (2017), who reported that their participants mainly agreed that information (e.g., raw data, diagrams) by activity tracking technologies are clearly presented.
Nevertheless, users within this sample tend to use diagrams a little less than raw data. The participants agree that they are using the diagrams to interpret and identify differences and saliences in their tracked data. As Gouveia et al. (2015) found out that their participants were in a short engagement phase, we assume that our participants also tend to have a quick look at the raw data instead of intensive reviewing and engaging with information by using diagrams (which include more detailed information). Furthermore, interpreting and identifying differences/saliences might also be useful if one considers greater amount of data over an extended period to compare those data with each other. This could be connected to the findings of Gouveia et al. (2015) that users were not interested in reflecting on data tracked in the past and, therefore, did not prefer the diagrams to the raw data. Reflecting on data tracked and interpreting or identifying these data are much more complex activities and need more time than gaining awareness regarding the current amount of steps or heart rate. The results also confirmed that the reason to buy an activity tracker does not need to be the same for everyone. As Rooksby et al. (2014) already mentioned, users have different goals such as documentation (e.g., awareness, reflection, confirmation) and diagnostic tracking (e.g., identification of connections between things, searching for an answer for situations). Participants in this study seem to tend to use it rather for documentary needs.
According to Hirvonen et al. (2012), we conclude that participants’ information needs and use in this study do not mainly require diagrams and that raw data seems already sufficient. The results confirm our assumption that diagrams are needed regarding higher task complexities and levels of activities such as interpreting and identifying differences and saliences as they provide detailed information instead of raw data. But they are also used for gaining awareness, improvement, and assessment of their own health and fitness-related behavior as well.
Even if most participants agree on interpreting differences and saliences, some participants disagree that the provided tracked sleep data is useful. This confirms the results by Maher et al. (2017) where a few participants did not know how to interpret or use the data. These results are also in line with Zhang et al. (2019), who call attention to the fact that wearables do not give suggestions. Therefore, users with higher task complexities are on their own. Diagrams could be interesting, but they might not be useful without knowing how to apply them. Users can try to interpret and to connect their insights with their environment and their behavior, but these insights are solely based on their own interpretation and knowledge. Therefore, it is also crucial to support users who want to gain added value from the diagrams and elaborate on their possibilities to improve health and fitness-related behavior. Especially if users might not have a solid knowledge about the heart rate zones, it is advisable to integrate the explanations into the diagrams as well. According to Patel and O’Kane (2015), users want to monitor their progress to measure and improve their exercising behavior. The users in this study mainly agree that they are also looking at their heart rate to improve it by making use of the diagrams.
6.2. Perceived Readability of Heart Rate Zones and Sleep Stages’ Characteristics (RQ2)
According to Nelson et al. (2016), the engagement with wearables and herewith provided information depends also on the readability. The participants’ evaluation is mainly positive as they agree that the information is not too long, easy to understand, and interesting. This is crucial, as the use of information can be affected negatively by the length. According to Case and Given (2016), information overload can lead to information avoidance. Therefore, activity tracking technologies must provide useful and user-friendly information to support and enhance the use of information. Furthermore, curiosity plays an important role as well, especially if the information has serendipity like in this survey. The survey’s design showed that users who saw the explanations for the first time consumed the information passively, as provided by the authors. Provided information needs to be interesting and at least useful; otherwise, the motivation to read information might be undermined.
6.3. Activity Tracking Technologies and Perceived Usefulness and Understandability of Provided Information (RQ3)
Regarding the health information behavior of the participants, there are further parallels to the study of Feng and Agosto (2019a). Users fulfill meta-level activities by using and thinking about the collected personal information, and by reflecting on those tracked data and evaluating it.
Concluding based on the results, both user groups mainly perceived the provided information as useful and easy to understand. They assess and make use of the explanations towards healthier behavior. Based on the explanations, they mostly agree that they already reflected on their behavior or adapted their behavior or would like to do this in the future. The explanations support users to better understand which heart rate zones they are exercising or to identify where optimization regarding sleep cycles is needed. Interestingly, explanations of the heart rate zones are highlighted in the application’s settings. The explanation is not directly linked to the charts and diagrams. Therefore, users need to know where the explanation is placed and that it exists. The sleep stages' characteristics explanations are integrated directly within the diagrams. Fitbit users do not need to search for it in the settings. We assume that this might also be one reason why many participants in comparison to sleep stages did not recognize the heart rate explanations and saw it for the first time during the survey.
Furthermore, most participants also agree that the information is necessary. The explanation of the heart rate zones enables insight regarding the meaning of each zone. Therefore, users have the chance to use these insights and to better decide if their training was satisfying. To understand if an exercise was successful, they must know if they would like to exercise in the cardio or fat-burning zone. Even if users mostly agree that they understand the explanations and know how to use the information—we would suggest involving experts (e.g., fitness coaches, doctors) to better identify differences and knowing how to use such insights.
The sleep stages explanation includes the sleep stages’ characteristics. But this does not help to fix the sleep behavior or to change it. Users must know how to change a specific behavior. First insights are a solid start (to gain awareness, to improve bedtimes and duration, to evaluate if there was enough sleep), but more is needed than an indication that the sleep quality is ‘bad’ or ‘good.’ Furthermore, we assume that changing behavior regarding the sleep stage explanation might be more complicated than to reflect on it. Here, users tend to be neutral about the statement that they adapted their behavior based on the sleep stage explanation. On the contrary, they mostly tend to agree that they reflected their behavior based on the explanation. This might also be an indicator that the complexity of reflecting on the behavior and adapting the behavior differs. One can reflect on data and think about it, but one still does not need to come to a conclusion on how to improve or change a behavior based on tracked data. Users need to understand the characteristics of the sleep stages and how they can change them. Therefore, this investigation confirmed equally like McKinney, Cox, & Sbaffi (2019) that tracking is an intensive activity.
7. LIMITATIONS AND CONCLUSION
The investigation concentrated on Fitbit users as a single case. It would be helpful to conduct further similar studies with users of other activity tracking technologies such as Garmin, Samsung Gear, or Apple Watch. This would enable a comparison regarding the presentation of explanations and their usefulness. Furthermore, most participants are exercising 1-2 or three or more times per week. This and the fact that half of the participants are long-term users might bias the results. Further studies need to concentrate on newbies and also on users who are exercising less. Furthermore, a limitation of this study is predefined answers. This allows only a limited insight regarding the information behavior of activity tracking technologies. Here we suggest that interviews would expand the understanding of information behavior in the context of activity tracking technologies in the future. Furthermore, we did not measure the level of health information literacy; rather, we asked for participants’ estimation if they are able to assess, use, and reflect on the data. Future investigations are needed to measure to what extent users of activity tracking technologies are health information literate. This could include investigations on aspects such as if they are seeking further information to better understand the explanations and, if so, how they seek further information, how they evaluate different obtained information and sources, and how they are synthesizing this information in detail.
This investigation ties in with previous studies and underlines the diversity and complexity of activity tracking technology studies. It is difficult to achieve a balance and to satisfy all kinds of users. As Case and Given (2016) mentioned, information overload can lead to stopping use of the information and, consequently, the device. In this study, the users mainly agree that the explanations were not too long. The question arises if users need maybe more detailed information regarding adapting the behavior. But results by Kinney, Nabors, Merianos, & Vidourek (2019) showed that there was no significant evidence towards a relation showing that the more information users are applying, the more they feel confident to be physically active. Nevertheless, our investigation shows that using two types of information (Type 1: raw data and Type 2: diagrams) provided by Fitbit satisfied the users’ information needs. Especially the explanations (Type 3) are characterized as necessary and easy to understand by most users. More to the point, users mainly agree that they know how to use obtained information by looking at explanations.
The improvement of one’s health and fitness-related behavior requires more than only acquiring information or gained knowledge. Activity tracking technologies might be motivators but not facilitators. First, users need the willingness to change or to recognize the need to improve health and fitness-related behavior. Second, users need the knowledge or ability to adapt knowledge to improve their behavior individually. The latter skill is not directly provided by visualizations of the collected data, as they do not contain suggestions on how to use specific observations. Fitbit already offers notifications regarding sleep quality, but these are general advocacies. Even if most participants agree that the tracked sleep data improves their sleep quality, there are also cases where users disagree. Regarding the diagrams, users get possibilities to compare themselves with average values. At this point, we stress that the solution is not to solely offer more information, but that it also needs to be useful and necessary. Users who are interested in improving their health and fitness-related behavior should get support through gyms and health insurance, as well as through educators in school or university.
References
Exploring context in information behavior: Seeker, situation, surroundings, and shared identities. In G. Marchionini (Ed.), Synthesis lectures on information concepts, retrieval, and services ( ) ((2018)) Morgan & Claypool Agarwal, N. K. (2018). Exploring context in information behavior: Seeker, situation, surroundings, and shared identities. In G. Marchionini (Ed.), Synthesis lectures on information concepts, retrieval, and services (pp. 1-163). Morgan & Claypool. , pp. 1-163
, Feng, Y., & Agosto, D. E. (2017, March 22-25). A survey on management of personal health information from activity trackers. , IConference 2017 Proceedings, (pp. 370-377). iSchools., , A survey on management of personal health information from activity trackers., IConference 2017 Proceedings, 2017, March 22-25, iSchools, 370, 377
, Fietkiewicz, K. J., Lins, E., Baran, K. S., & Stock, W. G. (2016, January 5-8). Inter-generational comparison of social media use: Investigating the online behavior of different generational cohorts. , Proceedings of the 49th Annual Hawaii International Conference on System Sciences (HICSS), (pp. 3829-3838). IEEE., , Inter-generational comparison of social media use: Investigating the online behavior of different generational cohorts., Proceedings of the 49th Annual Hawaii International Conference on System Sciences (HICSS), 2016, January 5-8, IEEE, 3829, 3838
, Fritz, T., Huang, E. M., Murphy, G. C., & Zimmermann, T. (2014, April 26-May 1). Persuasive technology in the real world: A study of long-term use of activity sensing devices for fitness. In M. Jones, P. Palnque, A. Schmidt, & T. Grossman (Eds.), , Proceedings of the SIGCHI Conference on Human Factors in Computing Systems, (pp. 487-496). ACM., , Persuasive technology in the real world: A study of long-term use of activity sensing devices for fitness. In M. Jones, P. Palnque, A. Schmidt, & T. Grossman (Eds.), Proceedings of the SIGCHI Conference on Human Factors in Computing Systems, 2014, April 26-May 1, ACM, 487, 496
, Gouveia, R., Karapanos, E., & Hassenzahl, M. (2015, September 9-11). How do we engage with activity trackers? A longitudinal study of Habito. In K. Mase, M. Langheinrich, D. Gatica-Perez, H. Gellersen, T. Choudhury, & K. Yatani (Eds.), , Proceedings of the 2015 ACM International Joint Conference on Pervasive and Ubiquitous Computing, (pp. 1305-1316). ACM., , How do we engage with activity trackers? A longitudinal study of Habito. In K. Mase, M. Langheinrich, D. Gatica-Perez, H. Gellersen, T. Choudhury, & K. Yatani (Eds.), Proceedings of the 2015 ACM International Joint Conference on Pervasive and Ubiquitous Computing, 2015, September 9-11, ACM, 1305, 1316
, Harrison, D., Marshall, P., Bianchi-Berthouze, N., & Bird, J. (2015, September 9-11). Activity tracking: Barriers, workarounds and customisation. , Proceedings of the 2015 ACM International Joint Conference on Pervasive and Ubiquitous Computing, (pp. 617-621). ACM., , Activity tracking: Barriers, workarounds and customisation., Proceedings of the 2015 ACM International Joint Conference on Pervasive and Ubiquitous Computing, 2015, September 9-11, ACM, 617, 621
, Ilhan, A., & Henkel, M. (2018, January 3-6). 10,000 steps a day for health? User-based evaluation of wearable activity trackers. , Proceedings of the 51st Hawaii International Conference on System Sciences, (pp. 3376-3385). HICSS (ScholarSpace)., , 10,000 steps a day for health? User-based evaluation of wearable activity trackers., Proceedings of the 51st Hawaii International Conference on System Sciences, 2018, January 3-6, HICSS, 3376, 3385
Mann-Whitney U Test using SPSS statistics. (Laerd Statistics) ((2018), Retrieved August 28, 2020) Laerd Statistics. (2018). Mann-Whitney U Test using SPSS statistics. Retrieved August 28, 2020 from https://statistics.laerd.com/spss-tutorials/mann-whitney-u-test-using-spssstatistics.php. , from https://statistics.laerd.com/spss-tutorials/mann-whitney-u-test-using-spssstatistics.php
, Li, I., Dey, A., & Forlizzi, J. (2010, April 10-15). A stage-based model of personal informatics system. In E. Mynatt, G. Fitzpatrick, S. Hudson, K. Edwards, & T. Rodden (Eds.), , Proceedings of the SIGCHI Conference on Human Factors in Computing Systems, (pp. 557-566). ACM., , A stage-based model of personal informatics system. In E. Mynatt, G. Fitzpatrick, S. Hudson, K. Edwards, & T. Rodden (Eds.), Proceedings of the SIGCHI Conference on Human Factors in Computing Systems, 2010, April 10-15, ACM, 557, 566
, Liang, Z., Ploderer, B., & Chapa-Martell, M. A. (2017, May 23-26). Is fitbit fit for sleep-tracking?: Sources of measurement errors and proposed countermeasures. In N. Oliver, M. Czerwinski, & A. Matic (Eds.), , Proceedings of the 11th EAI International Conference on Pervasive Computing Technologies for Healthcare, (pp. 476-479). ACM., , Is fitbit fit for sleep-tracking?: Sources of measurement errors and proposed countermeasures. In N. Oliver, M. Czerwinski, & A. Matic (Eds.), Proceedings of the 11th EAI International Conference on Pervasive Computing Technologies for Healthcare, 2017, May 23-26, ACM, 476, 479
Fitbit - Statistics & facts. ( ) ((2019), Retrieved August 28, 2020) Liu, S. (2019). Fitbit - Statistics & facts. Retrieved August 28, 2020 from https://www.statista.com/topics/2595/fitbit/ , from https://www.statista.com/topics/2595/fitbit/
, Lupton, D. (2014, December 2-5). Self-tracking cultures: Towards a sociology of personal informatics. In T. Leong (Ed.), , Proceedings of the 26th Australian Computer-Human Interaction Conference on Designing Futures: the Future of Design, (pp. 77-86). ACM., , Self-tracking cultures: Towards a sociology of personal informatics. In T. Leong (Ed.), Proceedings of the 26th Australian Computer-Human Interaction Conference on Designing Futures: the Future of Design, 2014, December 2-5, ACM, 77, 86
, Patel, M., & O’Kane, A. A. (2015, April 18-23). Contextual influences on the use and non-use of digital technology while exercising at the gym. In B. Begole, J. Kim, K. Inkpen, & W. Woo (Eds.), , Proceedings of the 33rd Annual ACM Conference on Human Factors in Computing Systems, (pp. 2923-2932). ACM., , Contextual influences on the use and non-use of digital technology while exercising at the gym. In B. Begole, J. Kim, K. Inkpen, & W. Woo (Eds.), Proceedings of the 33rd Annual ACM Conference on Human Factors in Computing Systems, 2015, April 18-23, ACM, 2923, 2932
, Rooksby, J., Rost, M., Morrison, A., & Chalmers, M. (2014, April 26-May 1). Personal tracking as lived informatics. In M. Jones, P. Palnque, A. Schmidt, & T. Grossman (Eds.), , Proceedings of the SIGCHI Conference on Human Factors in Computing Systems, (pp. 1163-1172). ACM., , Personal tracking as lived informatics. In M. Jones, P. Palnque, A. Schmidt, & T. Grossman (Eds.), Proceedings of the SIGCHI Conference on Human Factors in Computing Systems, 2014, April 26-May 1, ACM, 1163, 1172
“It only tells me how i slept, not how to fix it”: Exploring sleep behaviors and opportunities for sleep technology. In N. Taylor, C. Christian-Lamb, & M. Martin, B. Nardi (Eds.), Information in contemporary society ( , , , ) ((2019)) Cham: Springer Zhang S., Schaub F., Feng Y., & Sadeh N. (2019). “It only tells me how i slept, not how to fix it”: Exploring sleep behaviors and opportunities for sleep technology. In N. Taylor, C. Christian-Lamb, & M. Martin, B. Nardi (Eds.), Information in contemporary society (pp. 754-766). Cham: Springer. , pp. 754-766