Introduction
The habitat of organisms is crucial for characterizing their life history and can influence the distribution, abundance, and survival rate of species (Potts et al., 2020). Of the many reasons, human-caused habitat loss or fragmentation significantly risks the species’ survival; therefore, understanding appropriate habitat conditions and the variables that influence the species’ habitat is crucial for conserving threatened species (Ramírez-Delgado et al., 2022). From an ecological perspective, environmental predictors can limit the spread of organisms and alter habitat suitability (Fournier et al., 2017). Ecological niche models, which use distribution points and environmental variables, are effective methods for estimating habitat suitability, the distribution of species, and the impact of environmental predictors (Regos et al., 2021).
The black-necked crane (Grus nigricollis) is a medium-sized crane, the only crane species in alpine habitats, which is endemic to the highlands of Central Asia on the Tibetan Plateau (Han et al., 2017). Owing to the declining global population of black-necked cranes, the species is currently classified as “vulnerable” by the International Union for Conservation of Nature (IUCN) (Birdlife, 2017). Black-necked cranes serve as flagship species in alpine wetland habitats and are regarded as environmental indicators (Li & Li, 2005; Niemi et al., 1997). Apart from China, in India, eastern Ladakh is the only known nesting habitat for black-necked cranes. Its population is estimated to be 80-100 birds in eastern Ladakh’s Changthang meadows, with a global population of approximately 10,070-10,970 birds (Birdlife, 2017).
Despite the decrease in the number of black-necked cranes, there is a lack of research on their habitat status, such as their spatial distribution, owing to extreme and harsh climatic conditions in Ladakh, India. Species distribution modeling is frequently used to forecast the geographical distribution of species at various spatial scales (Elith et al., 2006; Guisan et al., 2017; Kaul et al., 2022; Singh et al., 2009; 2020). Ecological niche modeling tools based on maximum entropy (MaxEnt), which is a machine learning technique, have been widely employed to make predictions to support conservation decisions (Addison et al., 2013). These tools rely on presence-only data to predict the potential spatial distribution of species using ecological and environmental variables. They have been used to improve the assessment of rare species and propose effective species conservation policies (Deomurari et al., 2023; Han et al., 2017; 2018). In the present study, we employed the MaxEnt modeling approach in an aim to predict the spatial distribution of black-necked cranes in the Trans-Himalayan region and identify conservation gaps as well as prospective breeding habitats to aid the long-term survival of the species.
Materials and Methods
Study area
Ladakh is a Trans-Himalayan landscape bordering Tibet (Fig. 1). The landscape is highly undulating with many open sandy plains, cliffs, and exposed rocks. Black-necked cranes are distributed across the Changthang area in eastern Ladakh, which contains a large number of wetlands (Chandan et al., 2006). The area covers approximately 27,000 km2 and has elevations ranging from 3600 to 7000 m above mean sea level (amsl). This region is an important highland grazing system in cold, arid deserts. The majority of Ladakh wetlands are glacial in origin and remain frozen between December to March. This area is inhospitable because of the strong and unpredictable winds, where temperature ranges from 0°C to 30°C in the summer and from –10°C to –40°C in the winter (Chandan, 2015). Precipitation majorly occurs in the form of snowfall, with an annual rainfall of less than 100 mm. The area also harbors several brackish and freshwater wetlands ranging in size from 0.01 to 120 km2 (Chandan, 2015).
Presence records
We downloaded the black-necked crane detection data from the Global Biodiversity Information Facility ( https://www.gbif.org/; accessed on August 23, 2022). Species data records were compiled in the form of GPS coordinates (DD, MM, and SS) obtained by citizens via surveys and other published data sources. Out of 1,085 black-necked crane GPS coordinates, we chose 486 GPS coordinates for modeling to avoid spatial overlapping. We obtained coordinates for each data point from the literature or using Google Earth 5.0.1 with geographic projection. We assumed that the geographic coordinates assigned to the records had positional accuracies of 20-50 m. We selected a geographical resolution of 1×1 km for locations corresponding to the environmental data (WorldClim).
Climate data
We used bioclimatic environmental variables from the WorldClim database (Hijmans et al., 2005). These metrics, derived from monthly temperature and rainfall climatology, are ecologically significant variables. We used 24 environmental variables (11 temperature and eight precipitation variables) and four variables were retrieved from an altitude with a resolution of 30 arcsecs, covering approximately 1 km2 area (Hijmans & Graham, 2006; Hijmans et al., 2005). The data layers of slope, aspect, and terrain roughness were created using the altitude data. Land Use Land Cover (LULC) data were downloaded from GlobCover V2.2 (ESA Globcover Project), a worldwide land use dataset with a spatial resolution of 1 km2 that has thematic LULC of 22 classes (Hansen et al., 2000).
Predicting spatial distribution
MaxEnt software (version 3.4.1 e; Phillips et al., 2017; http://biodiversityinformatics.amnh.org/open_source/maxent/; acessed on September 6, 2022) was used to predict the spatial distribution of black-necked cranes using a maximum entropy approach to calculate the probability distribution (Elith et al., 2011; Phillips et al., 2006). Owing to the small sample size, we implemented simple linear and quadratic features with a maximum of 10,000 background points (Phillips & Dudik, 2008). All the other default values were retained. For model development, we conducted 100 iterations (Singh et al., 2020) and randomly segregated the occurrence sites into two sub-samples, utilizing 75% of the locations as the training dataset and the remaining 25% for testing the developed models. The accuracy of the model was measured using the area under the curve (AUC) of a receiver operating characteristic plot (ranging from 0.5=random to 1=perfect discrimination); furthermore, the relevance of the variables was determined using the jackknife approach (Yang et al., 2013). We calculated a likelihood map of the presence of black-necked cranes by averaging 100 model predictions. We obtained the shape files of protected area (PA) boundaries of each state from the World Database on Protected Areas (WDPA) (IUCN and UNEP 2009) to assess the suitability of habitats within and outside them. The potential spatial distribution map of black-necked cranes was estimated with the habitat suitability index of “0” to “1” derived using MaxEnt. The probability of spatial distribution was categorized into four grades with HSI: the area with 0-0.2 HSI was estimated as unsuitable habitat, 0.2-0.4 HIS as low habitat suitability, 0.4-0.6 as moderate habitat suitability, and 0.6-1 as high habitat suitability.
Results
Our MaxEnt model had a high AUC score (standard deviation, SD) for the training data (0.98±0.002 SD) with a test AUC score of 0.966 (models with AUC scores >0.75 are deemed potentially useful and have great predictive performance). According to the jackknife evaluation technique, 14 variables contributed to 96.2% of the model predictions (Table 1). The jackknife test revealed that precipitation (Bio12, Bio14, Bio15, Bio18, and Bio19), temperature (Bio2, Bio3, Bio4, Bio5, Bio8, and Bio9), and topography (Terrain ruggedness and altitude) were amongst the most important variables that accurately predicted the spatial distribution of black-necked cranes in the Trans-Himalayan region (Table 1; Supplementary Fig. 1). The temperature-based variables had the most influence (43.2%) on the model, while precipitation- and topographic-based variables contributed 32% and 23.4%, respectively, predicting the black-necked crane distribution; however, the LULC-based variables had no effect on the model (Table 1). According to our model projections, the likelihood of predicting the spatial distribution of black-necked cranes decreased as the temperature in summer rose beyond 25°C (Fig. 2A), the terrain roughness increased (Fig. 2B), and the variation in seasonal precipitation increased (coefficient of variation=30%) (Fig. 2C). The model predicted the geographical distribution of black-necked cranes in the Trans-Himalayan region of Ladakh with a low suitability threshold (Fig. 3). We anticipated the geographic area distribution of black-necked cranes as 8,156 km2, 6,759 km2, and 36,414 km2 with high, moderate, and low potential spatial distribution, respectively. Within the PA (9,000 km2), the area with high habitat suitability was estimated to be 5,335 km2 (60.3% of PA).
Discussion
To analyze the potential distribution of black-necked cranes in Ladakh, in the Trans-Himalayan region of India, we used the maximum entropy model along with the data acquired by the local citizens. According to the ecological niche theory, the distribution of a species is largely influenced by specific environmental requirements and spatial heterogeneity (Chase & Leibold, 2003). Consequently, the environmental variables used for evaluating habitats of black-necked cranes were confined to climate and terrain, which played an important role in identifying spatial distribution and habitat appropriateness of this species. The current distribution range of breeding black-necked cranes projected by the model is primarily centered in the eastern half of the Ladakh-Changthang region of the Trans-Himalayas (Chandan et al., 2014). The regions with environmental conditions similar to the occurrence locations across the species distribution range were identified using MaxEnt models (Pearson et al., 2007). However, the population is smaller and more dispersed. Moreover, breeding occurs at heights ranging from 2,950 to 4,900 m on the Qinghai-Tibetan Plateau and from eastern Ladakh of India to the northern Sichuan province of China.
When compared to succeeding predictions, our model accurately predicts the spatial distribution of black-necked cranes based on temperature and terrain ruggedness, which outweighed the other 22 environmental factors studied here. As shown earlier, temperature had a greater impact than precipitation, according to the performance of bioclimatic predictors (Li & Li, 2005). The crane breeding season primarily falls during the warmest months of the region (Han et al., 2017). The temperature fluctuations control the majority of important ecological factors, including summer soil water content (Liu et al., 2012), biological moisture availability, and the length and distribution of plant growing seasons, all of which affect the suitability of the environment (particularly the availability of food) for breeding cranes (Li & Li, 2005). Apart from China, the only known crane breeding population is in the eastern region of Ladakh, India. Black-necked cranes were bred in Ladakh in late March and early April. According to a field study, breeding black-necked cranes favor wetlands near lakes (Chandan et al., 2014). In the Changthang region, aquatic plants thrive in the shallow areas of wetlands in summer, and sedges dominate the stream banks and surrounding marsh meadows (Rawat & Adhikari, 2005). These wetland habitats have been shown to be potential nesting grounds for black-necked cranes as well as for a number of other migratory and resident bird species.
Black-necked cranes are at risk of going extinct because of the increased livestock breeding in summer pastures, which have decreased snow and ice. There has been a shift in the spring arrival and departure dates of migratory birds because of climate change. The newly identified suitable habitat may turn into a migratory stopover for many black-necked cranes and other waterfowls, as migratory birds have been shown to respond to changes in environmental resources. Therefore, climate change may cause an earlier migration of black-necked cranes, which has also been reported for other crane species (Zhang et al., 2012).
The Changthang region of eastern Ladakh, which serves as a crane nesting area, has a conservation gap that makes it difficult to draw useful conclusions. The spatial distribution predicted in this study shows that the majority of estimated conservation areas bordered the existing protected areas of the Changthang Wildlife Sanctuary (CWLS). By increasing the current CWLS area, these conservation gap areas can be covered more effectively. To date, studies of black-necked cranes during the breeding season have mostly included descriptive observations of their status in a few areas and the effect of dogs on nesting birds. As a result, our findings can significantly aid in developing conservation and management strategies for blacked-necked cranes in this area. To expand the limits of the CWLS, a base map based on the highly suitable habitat areas identified here should be adopted. Habitat blocks enable species to grow and may change in response to climate change, in the future. Overall, our research reveals the potential habitat of black-necked cranes and identifies plausible steps to protect this threatened species from getting extinct.
References
BirdLife International (2017, Retrieved Apr 13, 2023) Grus nigricollis. The IUCN Red List of Threatened Species from http://www.iucnredlist.org/details/22692162/0
, , (2017, Accessed on 2022-September-06) Maxent software for modeling species niches and distributions (version 3.4.1), http://biodiversityinformatics.amnh.org/open_source/maxent/
, (2005) Floristics and distribution of plant communities across moisture and topographic gradients in Tso Kar Basin, Changthang Plateau, Eastern Ladakh Arctic, Antarctic, and Alpine Research, 37, 539-544 https://doi.org/10.1657/1523-0430(2005)037[0539:FADOPC]2.0.CO;2.
Figures and Table
Fig. 2
(A-H) Relationships between top environmental predictors and the probability of occurrence of black necked cranes in Ladakh region of trans Himalaya, India.
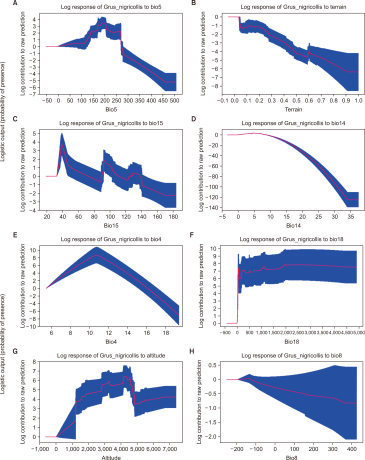
Fig. 3
Predicting spatial distribution of black necked cranes in Ladakh region of trans Himalaya, India.

Table 1
Variables used in the predicting the potential distribution of black necked cranes in trans Himalayan Region, India
Code | Environmental variables | % Contribution | Permutation importance |
---|---|---|---|
Bio5 | Max temperature of warmest month | 27.5 | 8.9 |
TRugd | Terrain ruggedness | 17.6 | 1.4 |
Bio15 | Precipitation seasonality (coefficient of variation) | 13.8 | 9.8 |
Bio14 | Precipitation of driest month | 7.6 | 5.2 |
Bio4 | Temperature seasonality (standard deviation ×100) | 7.3 | 6.3 |
Bio18 | Precipitation of warmest quarter | 5.3 | 3.6 |
Alt | Altitude | 4.5 | 19.5 |
Bio8 | Mean temperature of wettest quarter | 3.6 | 0 |
Bio12 | Annual precipitation | 2 | 3.4 |
Bio9 | Mean temperature of driest quarter | 1.9 | 1.1 |
Bio3 | Isothermality (P2/P7) (100) | 1.5 | 2.2 |
Bio11 | Mean temperature of coldest quarter | 1.3 | 1.4 |
Bio19 | Precipitation of coldest quarter | 1.2 | 8.5 |
Bio2 | Mean diurnal range (mean of monthly [max temp-min temp]) | 1.1 | 0.1 |
Slope | Slope | 0.8 | 0.4 |
Bio1 | Annual mean temperature | 0.7 | 5.1 |
Bio7 | Temperature annual range (P5-P6) | 0.6 | 0.2 |
Aspect | Aspect | 0.5 | 0.1 |
Bio10 | Mean temperature of warmest quarter | 0.3 | 6.2 |
Lulc | Land use/land cover | 0.3 | 0.2 |
Bio17 | Precipitation of driest quarter | 0.2 | 4.2 |
Bio16 | Precipitation of wettest quarter | 0.2 | 1.8 |
Bio6 | Min temperature of coldest month | 0.1 | 3.5 |
Bio13 | Precipitation of wettest month | 0.1 | 6.7 |